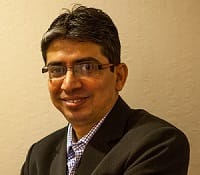
By Subhasis Bandyopadhya, Head of BFS Practice, Mindtree
As businesses of all sizes continue on their journey of digital transformation, the adoption of technology continues to grow. The financial services industry has been particularly receptive to these new innovations; however, these advances have not been without the emergence of ongoing challenges along the way.
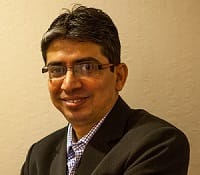
Subhasis Bandyopadhya
With digitisation taking hold at an ever-growing rate, banks and financial services institutions alike have also increasingly been tasked with fighting financial crime, money laundering and the funding of criminal activity.
The growing tendency for banks to transfer funds electronically has been one such trend amidst this ongoing digitisation process. As a result, financial services institutions have been forced to continually monitor and detect any suspicious activity, with banks being required to have procedures in place to prevent this kind of activity from materialising in the first place.
To successfully adapt to this changing financial landscape, they must adopt the latest tools with in-built self-learning capabilities – or risk falling behind the competition.
As regulators have stepped up their levels of scrutiny, particularly in transaction monitoring, those banks that fail to comply risk facing substantial fines. A recent example was that of the Commonwealth Bank who allegedly failed to report disclose details of $77m worth of suspicious transactions to the Australian financial intelligence agency, Austrac. The bank is now facing legal proceedings for serious and systematic violations of anti-money-laundering and counter-terrorism laws.
Out with the old
Sometimes technology doesn’t always work the way we want it too. In the case of traditional monitoring systems however, this has become the expected norm despite banks ongoing reliance on said processes to detect suspicious transactions.
For example, setting up the appropriate threshold levels and parameters for alert detection has proven to be a persistent problem. If thresholds are set too low, the system will populate itself with a high number of alerts, all of which require analysis. However, if these thresholds are set too high, the amount of alerts will decrease as a result, meaning the company may be unable to detect all suspicious activities. This means the risk of failure to meet regulatory requirements, and subsequent reputational damage or financial exposure becomes very real indeed.
The identification of false positives in data, quickly and accurately, is also an all too evident issue. It is critical that these alerts are not only analysed carefully, but also in a timely manner. Taking action on false positives at the right time, and removing them as quickly as possible, is likely to be one of the biggest challenges facing banks and financial services firms in the technology space.
These implementation issues, combined with the need to comply with global regulations and streamline operations to minimise costs, are complications that are just too great for humans to overcome alone.
In with the new
Adopting machine-learning and artificial intelligence (AI)techniques are the solutions to the woes inflicted by transaction monitoring. By leveraging machine-learning powered predictive analytics platforms, which can continuously evolve with new data points and user analysis, this will fortify those established decision support systems.
Given their highly accurate decision-making abilities, these intelligence platforms also provide banks and financial services institutions with detailed audit features, allowing them to improve predictions and drive operational efficiency.
Machine-learning and AI have also been successful in identifying significant spikes in the value or volume of transactions; monitoring high risk jurisdictions, identifying the rapid and unusual movement of funds, screening both sanctioned and politically exposed individuals, as well as monitoring for activity from known terrorist organisations.
Thanks to such repetitive analysis, these developments will benefit banks and financial services immensely from a security standpoint.
Moving away from traditional rule-based models to dynamic predictive models will allow banks to perform real- time, transaction-based detection techniques. Machine learning platforms can create dynamic workflows and have built in self-learning capabilities capable of identifying those transactions that represent genuine risks. The platforms can also have the ability to carry out accurate confidence scores for enhanced business functions and fast deployment time to market built in, in advance.
It is not only the industry experts that are realising the full potential of this technology, but regulators too are coming round to the view that mere rules alone are not an effective means of detection and prevention. Regulatory boards are encouraging financial services institutions across the board to adopt these new technologies in order to detect anti-money laundering and unearth any suspicious activity before it comes to pass.
Looking ahead
We are currently witnessing a sea of change in contemporary banking. Robust regulatory demands and the drive to digitise have meant that the financial services landscape is facing a set of challenges on a previously unseen scale.
For established banks to not only survive, but thrive, in this new digital age, then it is crucial that the industry as a whole embraces machine-learning and AI technologies in the transaction monitoring process.
The departure from traditional rule-based systems and the adoption of cutting-edge new platforms will not only cause banks to substantially reduce operational costs, but process large amounts of data seamlessly, improve alert predictions, and deliver accurate results day-after-day. Collectively this will be key to keeping regulators globally at bay.