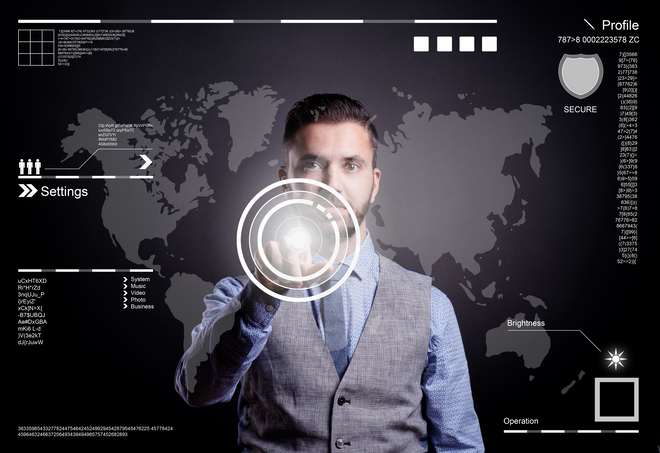
By Antony Bream, Global Head of Enterprise Sales, AimBrain
In the four years since AimBrain was born, we have seen a meteoric rise in the capabilities of today’s wider biometric authentication modules, but similarly a staggering rise in the sophistication of fraud capabilities. No longer opportunities or curious hackers looking to exploit bugs for fun, today’s fraud rings are as capable and organised as legitimate enterprises
You have been pwned
Credential breaches are reaching staggering figures; this month both Quora with 100 million users breached and Marriott with 500 million users’ personal information including passport details and in some cases credit card information…there is no doubt about it, it is extremely unlikely that you have not been breached. Worryingly, even those who have been breached continue to use passwords that they know to have been stolen, one study showed 86% of Cashcrate subscribers continued logging in with passwords already leaked in other data breaches.
Can we take it all back?
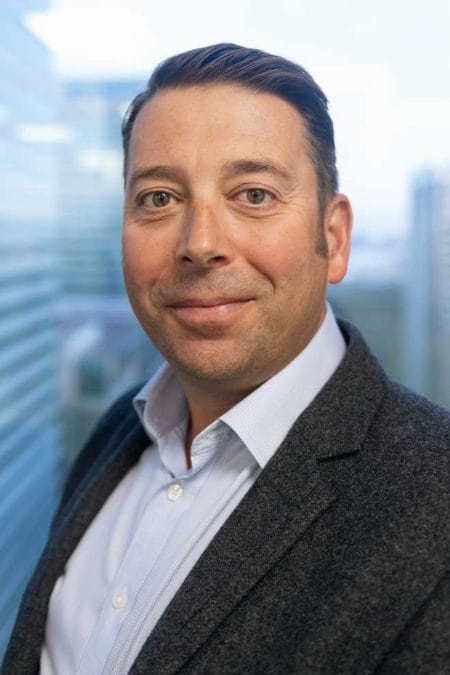
Antony Bream
So how do we ‘unfeed the data dragon’ and reclaim our personal data that is in the public domain; copies of passports, scans of birth certificates, medical records, bank statements? How do we ensure that it’s not our information being sold on the dark web; name, social security/national insurance number, last known address?
We can’t. Which makes it all the harder to counter an increasingly smart synthetic identity business. Using stolen, fraudulent and real identities, or combinations thereof, criminals are now applying for credit cards, bank accounts and other financial services open to them. Mule accounts assist the quick dissemination of money gathered through illicit means, often making the recuperation difficult or impossible, and at the mercy of the financial services organisation to repay.
A united front
I’m pleased to see that there is more and more consolidation of both data and experience in the industry, particularly around problems like mule accounts. Suspicious individuals and historic account behaviour is helping today’s banks identify dormant mule accounts and stop the abuse before it happens, with data sharing helping to paint wider and richer pictures of the fraud ecosystem.
Alongside this, machine learning and deep learning are increasingly being deployed in order to pinpoint specific behaviours attributed to criminals. The obvious indicators are well documented; fraudsters’ preferences for long weekends for example, or keystroke patterns for particular pieces of personal information. But deep learning is self-evolving, which means that it can now consume unprecedented volumes of data and spot patterns that humans could never hope to, continually refining to create ever more precise models.
These models can now be used at the new account opening stage in the fight against synthetic identity fraud, to be able to pinpoint patterns in manual fraud, not just protect against bots.
Anomaly detection as a first sweep
Using anomaly detection such as this, to identify fraud before a user’s profile exists, stops fraud from getting into an organisation and setting down roots. But what about those that seek to exploit legitimate customers? Account takeover or phishing attacks for example?
This is where other biometric authentication factors come in. Behavioural authentication for example continuously and passively authenticates a user’s behaviour – across any device with a keypad, touchscreen, mouse or keyboard – and monitors for signs of change. Too far away from the behavioural template, and a bank can invoke another security challenge; a password reentry or active authentication step like fingerprint or selfie.
Passive and active
Abnormal transactions or changes to personal information such as address, email or back-up phone numbers for example, could use a combination of passive and active steps. Behaviour authentication can invisibly be paired with device location or ID, and if either falls out of a risk tolerance levels, an active step be invoked demanding the user fulfil a particular activity such as a voice authentication or facial authentication.
What’s more, these authentication tools are more often than not delivered via APIs, which means that they slot easily into a bank’s risk engine. The bank can adjust its own decisioning trees for specific use cases, configuring passive and active biometrics as part of its wider multifactor authentication strategy. They work with risk scores to construct the risk models that suit the impact and likelihood of a breach, yet the complexity of the security is all but invisible to the end user.
It’s a bright future for algorithms
Furthermore, we’re seeing developments in deep learning to be able to learn from existing annotated data – data that has correctly been previously categorised as fraud for example. Whilst the financial services industry only has a finite amount of records, and whilst this can’t be scaled beyond the fraud that they’re capturing, our Machine Learning team are seeing more effort go into reusing data. One way is through transfer learning (training models on one task and using this as a starting point for different, typically more complex tasks), as well as unsupervised and self-supervised learning, models drawing inferences from unlabelled or uncategorised data, and prediction modelling using part-labelled data, respectively.
It’s an extremely exciting time for fintechs as financial services firms see the real-world benefits of machine learning in fraud detection. The pairing is complementary; AI-focused fintechs like ours can continue to focus on research and development for fraud detection, whilst banks can benefit from our solutions, and the modelling capabilities can continue to evolve to help solve the fraud of tomorrow.