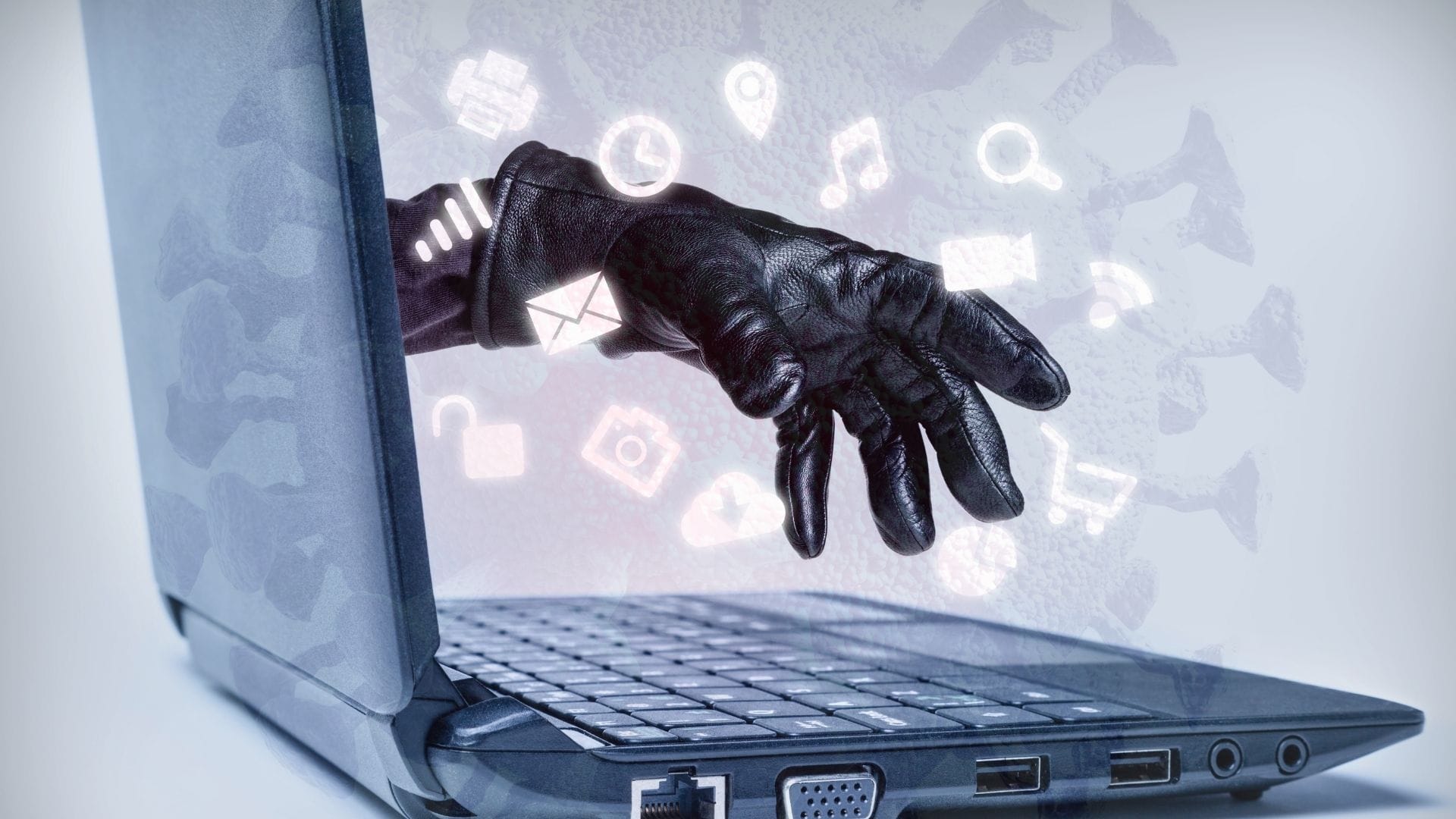
By Amy Hodler, Director of Analytics and AI Program at Neo4j
Coronavirus aid money has become a major target for cybercriminals. Graph database technology can uncover links between data that expose the fraudsters effectively, explains Neo4j’s Amy Hodler
Coronavirus has been a gift for cybercriminals and they have been quick to exploit it. With the pandemic moving so fast, and funds being issued so swiftly the potential for scams and fraud are huge. The World Health Organisation, for example, has seen a five-fold increase in cyberattacks since the pandemic started.
Bad actors are using fake identities to syphon off governmental emergency funds, for example. This behaviour is particularly severe in Germany, where fraudsters are trawling businesses applying for emergency funds. This data is used to divert funds from state accounts into their own fraudulent bank accounts. The government of German province North Rhine-Westphalia is believed to have lost tens of millions of euros in a recent phishing attack. Cybercriminals cloned an official website designed to distribute COVID-19 financial aid. Personal details provided by applicants on the site were used to fraudulently apply for funds and collect payments on their behalf.
In a joint advisory, the UK’s National Cyber Security Centre (NCSC) and the United States Department of Homeland Security (DHS) Cybersecurity and Infrastructure Security Agency (CISA) have issued a warning that a number of malicious cyber actors and advanced persistent threat groups are extremely active. They are targeting individuals, small and medium businesses and large organisations with COVID-19 scams and phishing emails.
In the financial services sector, companies continuously check and compare transactional and personal data to monitor for suspicious actions in the fight against fraud. Similar to the application for financial aid, bad actors that defraud financial institutions use false or synthetic identities when creating accounts or loan applications. Information such as home address, phone number and email details utilise identities that are reconstructed to create a totally fictitious persona. According to McKinsey, this results in major losses for financial institutions, with an estimated 80% of all credit card fraud losses stemming from synthetic identity fraud.
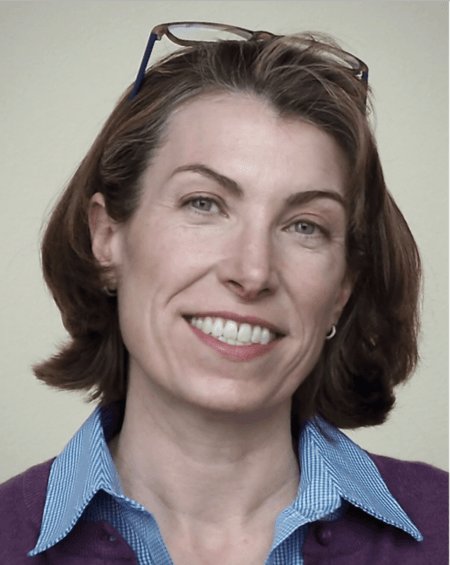
Amy Hodler
Conventional fraud detection solutions are not performant enough to uncover these synthetic identities. Such solutions can only relate two to three pieces of data at any one time, such as name, home address or bank account. This can be sufficient for trapping individual perpetrators, it just isn’t sophisticated enough to uncover fraud rings where multiple parties are involved.
Conventional approaches ineffective
The main reason why conventional approaches to fraud monitoring are so poor, is that the majority of fraud detection systems are based on a relational database model. This means that data is stored in a format of predefined tables and columns. With large, unstructured data sets, relational databases rapidly hit their limits. Queries end up being too convoluted and response times too protracted.
In addition, relational database solutions are trying to detect fraud with no real context. Banks and government authorities need the ability to monitor a trail from one account to another. This requires having a 360-degree view of the intricate complexity of the fraud network to determine how fraudulent activities are related.
Graph database technology may be an essential weapon in fighting bad actors. In contrast to relational databases, graphs not only interpret individual data such as person, account number and home address, but also their relationships with one another. This includes links such as ‘resident in’ or ‘transacted with’. The data model can thus accurately portray these complex relationships. Entities and relationships are referred to as ‘nodes’ and ‘edges’ or ‘relationships’.
The genius of graph database technology is that any number of qualitative or quantitative properties can be assigned, showing complex relationships in a coherent and descriptive way.
Mapping complex connections recoups billions of dollars
An example of the facility graphs have in uncovering hidden connections is highlighted by the International Consortium of Investigative Journalists, the group behind the Panama and Paradise Papers. The group used graph technology to map incredibly complex financial connections and spot irregularities. Graph technology has played a major role in recouping more than $1.2 billion in resulting fines and back taxes since the original investigation back in 2016.
Staying one step ahead of cybercriminals is hugely challenging in a time of unprecedented change. Graph technology can easily identify fraud rings and other scams with a very high level of accuracy. Uncovering connections between data in real time is key to ensuring coronavirus crisis funding reaches those in real need, and not the cybercriminal community.