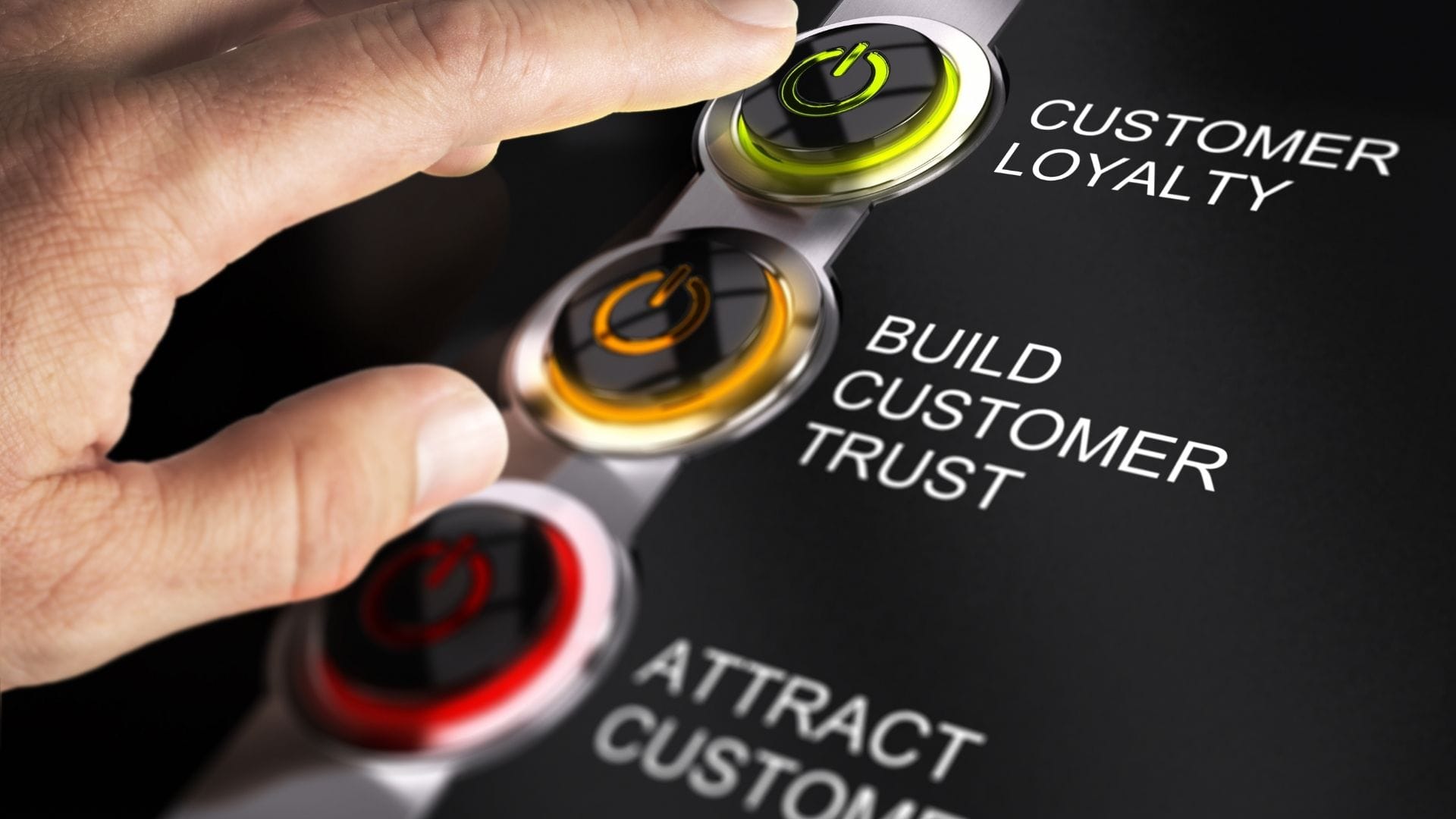
By Helena Schwenk, Market Intelligence Manager at Exasol
Financial interactions have drastically increased over digital channels due to COVID-19, with customer behaviour adapting to the restrictions of a pandemic, where economic constraints are in the spotlight and attitudes towards risk have changed. This makes an advanced data strategy more crucial than ever – particularly for financial services organizations, where the economic pressures are inherent.
Amidst the changes of the last eight months, one thing that remains the same is the importance of customer loyalty. Our survey of senior financial decision-makers found 80% consider loyalty a key priority, given its importance to revenue generation and differentiation from competitors.
If businesses can organise and analyse their customer data in a way that allows them to interpret and predict the behaviour of their customers, they will be able to extend their relationship with customers. Research from McKinsey supports this, suggesting that organisations need to rethink how they drive revenue to accommodate new consumer behaviours. This might mean new product offerings or repositioning offerings that are advisory and protection focused.
However, this is easier said than done in a sector that has always been data-intensive due to the breadth and depth of its customer touchpoints, with IDC conservatively predicting a 26% CAGR data growth in financial services organisations by 2025. That’s without considering how siloed and difficult to navigate financial data can be.
Navigating and interpreting this data demands a sophisticated data analytics strategy. Financial firms need an infrastructure that enables the real-time analysis of governed and operationalised data, integrating it business-wide while eliminating the unnecessary silos that slow down analysis.
Strategy and infrastructure
The first step in this process is to define the data analytics strategy, so that teams can extract all the insights they need from their organisation’s data. This is fundamental to understanding how to improve business operations, new services and products and, ultimately, customer lifetime value.
A robust data strategy also offers a view into customer retention, identifying clients at risk of being lost, with behavioural analytics providing the basis of personalised action plans. Improvement in these areas will deliver a more desirable customer experience and a much-improved understanding of customer loyalty.
This can only be beneficial to an organisation’s bottom line; according to Bain & Co., increasing the rate of customer retention by just 5% can increase profits by up to 95%.
The price of loyalty
Actioning an improvement in customer retention is not as simple as gathering insights. Loyalty is earned, and there are challenges to overcome.
For example, 54% of those we surveyed suggested that customers have higher expectations of financial services experiences than are being delivered on. 42% agreed that digital disruptors were encroaching on their customer base through new experiences and business models, while PSD2 and GDPR frameworks are impacting the ability of 41% to improve loyalty initiatives.
These challenges need to be tackled head-on because the risk of gambling on customer loyalty is too great. The business impact of poor customer loyalty is serious in terms of higher levels of customer churn (45%), lost opportunities for customer engagement (45%), and lost opportunities for revenue generation (42%).
It costs five times more to acquire a new customer than to sell to an existing one, and so these concerns are simply too high a set of risks to ignore.
Driving for improvement
The good news is that a more comprehensive understanding of customer lifetime and loyalty is possible. The foundations are already there in the majority of cases; 97% of organisations use predictive analysis as part of their loyalty initiative and customer insight processes, with 62% considering it a key component.
This high percentage is proof that data analytics is maturing in financial services. 96% are very positive about their firm’s data strategy and almost two thirds (65%) agree that data analytics enable them to predict the future behaviour of customers and offer personalisation.
Although, 48% believe that their data strategy could be improved, showing an appetite for progression. Things are improving, but many are not yet demonstrating their full potential.
What’s possible
One financial organisation fulfilling its data potential is Revolut. As one of the UK’s unicorn companies it is no stranger to explosive growth, with data volumes growing 20-fold within a 12-month period.
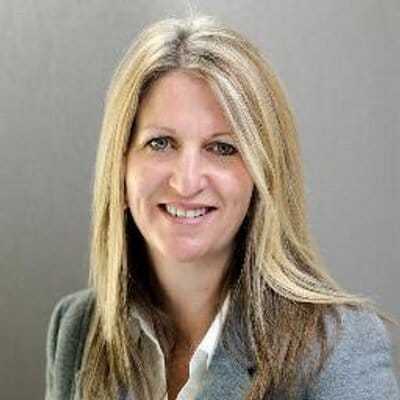
Helena Schwenk
Such growth made the maintenance of 800 dashboards and fielding over 100,000 SQL queries on a daily basis very challenging. To cope, Revolut transitioned to an in-memory data analytics database that suited its need for a flexible, hybrid cloud environment.
By acting as a central data repository, the database has saved time across multiple business units as is now able to resolve queries and reports in seconds instead of hours. According to Revolut’s data scientists these query times are now 100x faster than with their previous solution. This improves decision-making and allows Revolut to explore customer demographics, debit card statements, online and mobile transfers, and payment and point-of-sale data in almost real-time.
Moreover, every employee has access to this repository as a ‘single source of truth’. This data is available for interrogation too, with business intelligence (BI) tools and self-service access available to everyone as opposed to just the data scientists. Key performance indicators (KPIs) were based upon this data as well, standardising performance goals for everyone across the entire organisation.
As a result, Revolut has defined tens of thousands of micro-segmentations within its customer base, built ‘next product to purchase’ models that increase sales and customer retention, and delivered granular personalisation to over 13 million users.
Beating the competition
The same principle applies for traditional banks or young start-ups: offering quick, easy and satisfying services to customers to drive retention is paramount. By using data to identify and predict customer trends, organisations can learn where to differentiate themselves from competitors and deliver the right products and services at the right time.
Whether data is stored in the cloud, on-premise or both, the right data analytics database – backed by a committed and robust data strategy – is key in a post-COVID world. It must emphasise the optimisation of the collection, management and integration of data to empower users to be more informed and more efficient for business-wide benefits that drive loyalty and last.